How is AI in Loan Management Transforming the Lending Industry?
Are you looking to incorporate AI solutions into your lending workflows? This guide discusses the use cases of AI in lending, its impact, and adoption challenges to help you with loan origination processes.
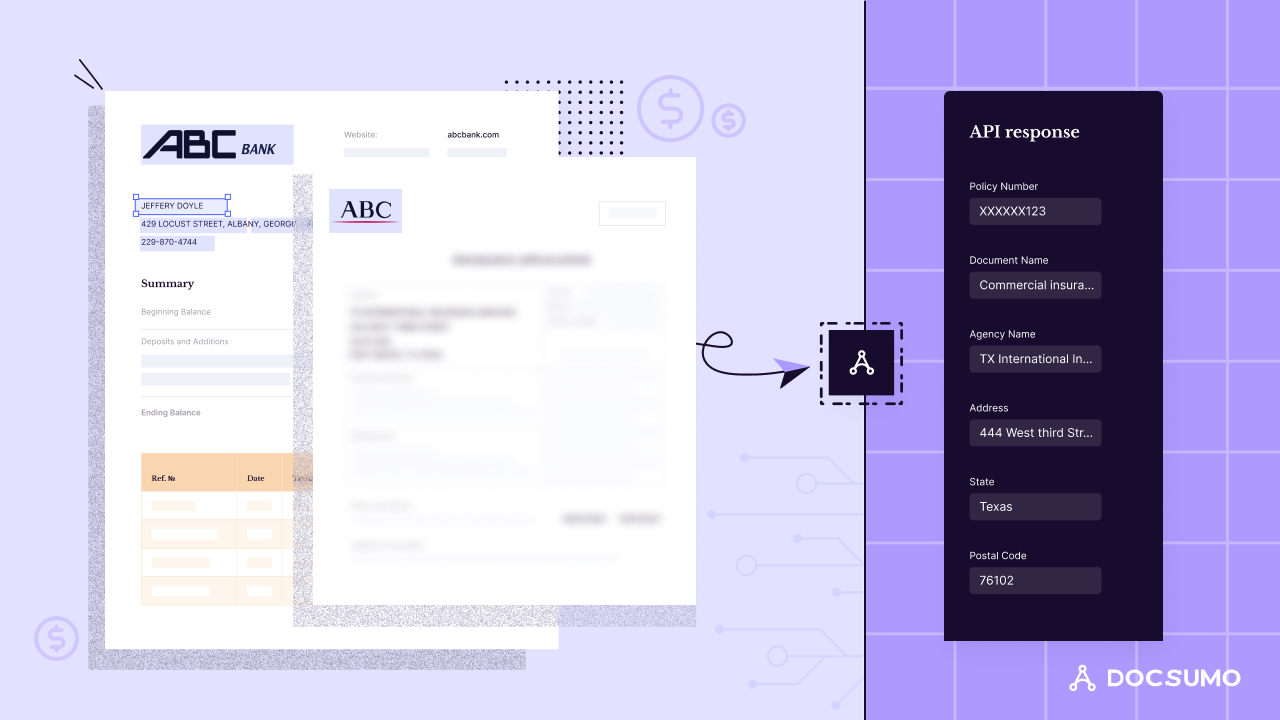
Banks and financial institutions face numerous challenges in loan sanctioning processes: heightened customer expectations, compliance requirements, an increased number of loan applications, and advancements in AI, which lead to nuanced threats.
According to BioCatch’s 2024 AI, Fraud, and Financial Crime Survey Report,
- 56% of fraud-management decision-makers at banks and financial institutions report financial crimes activity increased in 2023
- 69% say that criminals are more advanced at using AI for financial crime
Outdated legacy systems and non-standardized loan processing methods may not be the best ways to sanction loans in 2024. These methods include manually verifying the identity and financial documents, ensuring eligibility, and detecting fraud.
As a result, banks and lending institutions are increasing their investments in advanced technologies such as AI to expedite loan processing and combat fraud. The above report also shows that 94% of organizations use AI/ML technologies to understand lending risk, and 87% report AI has increased the speed with which their organization responds to potential threats.
This article discusses how AI is a must-have in the lending industry and the challenges in the adoption process.
What is AI in Lending?
The Global AI in lending market is expected to be worth around USD 58 billion by 2033, growing at a CAGR of 23.5% during the forecast period from 2024 to 2033. Several factors, such as automation, reduced operational costs, streamlined workflows, and a data-driven approach, are driving the adoption of AI in the lending industry.
For instance, this transformative technology automates tasks such as scanning borrower's documents, analyzing spending patterns and creditworthiness, and detecting fraud and manipulations. This automated process offers several benefits for lending businesses:
- Reduces lengthy loan processing and approval time
- Detects fraud efficiently
- Reduces human errors
AI and ML algorithms analyze vast amounts of data, investigate income and identity data, and detect tampering. This aids decision-making and enhances precision in loan approval processes. The result? Borrowers and customers will experience a more seamless and accurate loan approval process, improving customer satisfaction and retention.
4 Use-Cases of AI in Lending
AI has been enhancing the capabilities of various workflows and systems across the finance industry.
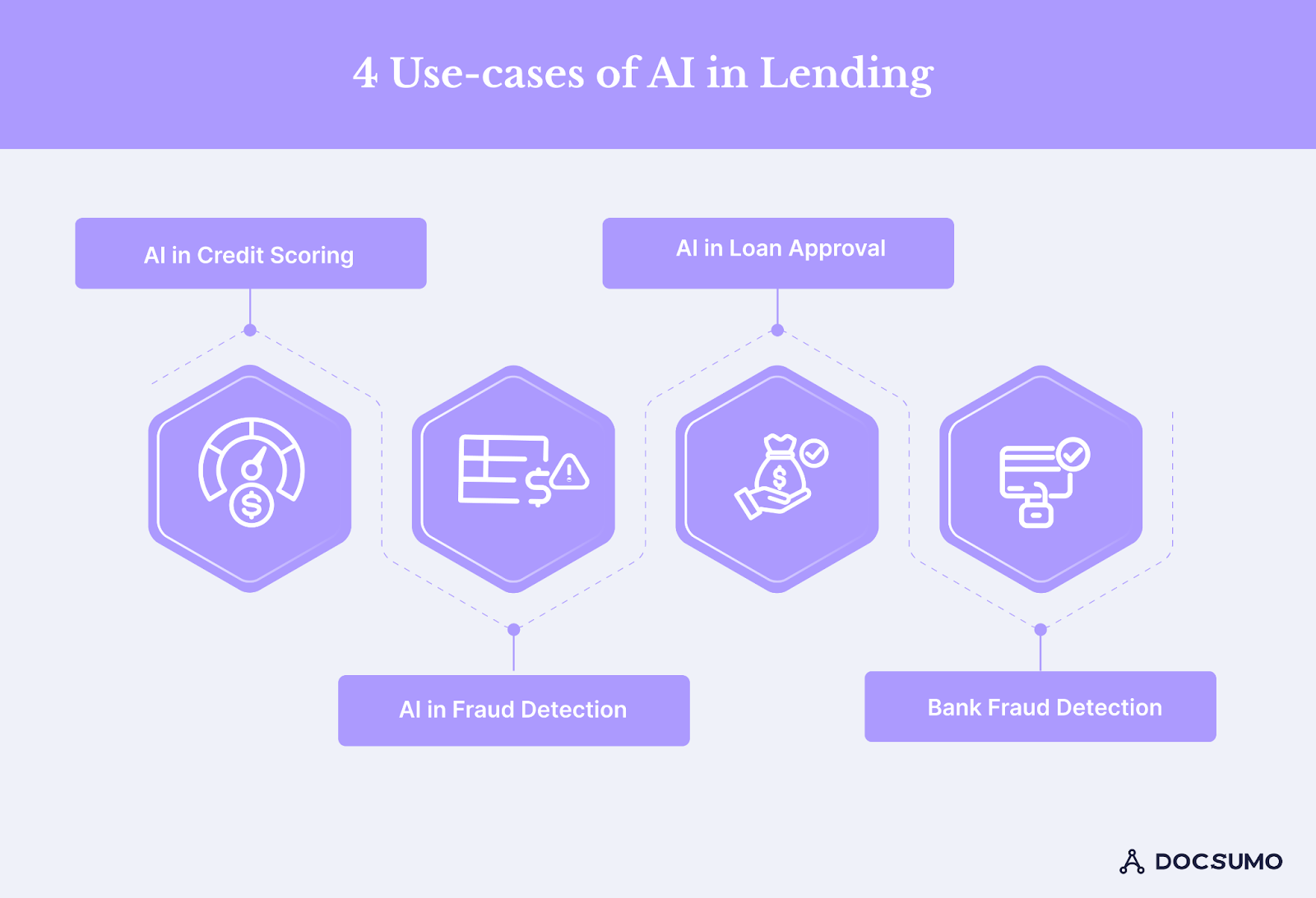
Specifically in lending, its use case extends to credit scoring, loan approval, fraud detection, collection management, and more.
1. AI in Credit Scoring
A credit score is evaluated by analyzing borrowing history, total income, work experience, and user behavior. The system gauges the user’s borrowing and generates a scorecard.
However, the traditional method of calculating the credit score has its fair share of drawbacks.
- To begin with, credit score is based on the customer’s historical financial data.
- New customers often need help securing loans even when they are creditworthy.
- Next, a credit score is inapplicable for new credit products created for the younger customer base.
- It makes the financial institution short-sighted and increases the risk of making errors in their credit estimation.
- Credit scoring models powered by machine learning and credit risk analysis monitor more relevant metrics, such as current income level, potential earnings, and employment opportunities, to measure new customers' creditworthiness.
In short, the AI loan credit scoring system increases financial inclusion by offering credit access to a previously unattended group of people.
2. AI in Loan Approval
The traditional loan approval process is exhaustive and resource-consuming. The loan officer collects documents, such as government-issued IDs, bank statements, employment proofs, salary slips, property papers, and other relevant documents.
After compiling all the documents, critical information is manually verified. After verification, getting the manager's final approval could take days, if not weeks.
AI-powered document processing software compiles specific information from the relevant documents at scale. It also checks the authenticity of submitted documents and routes applications through the respective departments for approval. The result is quicker loan approval times and an improved customer experience.
3. AI in Fraud Detection
According to recent reports, an average American bank faces 24,000 fraud attacks yearly. Unauthorized transactions and phishing scams are the leading reasons for increased attacks. However, identity theft and misrepresentation of financial statements are the root causes of loan fraud.
AI loan tackles fraud detection with four solutions: fraud scores, fraud investigation, purchase profiles, and KYC.
Bank Fraud Detection
Faking transactions and identities has become easy today. Deloitte’s Center for Financial Services report shows that Generative AI is expected to increase the threat of fraud, which could cost banks and customers as much as USD 40 billion by 2027.
AI-powered document processing software helps banks reduce the workload of banking staff by automating the document analysis processes. AI algorithms effectively analyze transactions and payments to identify suspicious activities and alert respective personnel to investigate further.
Fraud scores
Lenders use fraud scores to measure the legitimacy of a transaction. NLP and ML analyze data such as past transactions, fraud accidents, and risk parameters the lender sets and awards a fraud score.
The current transaction is flagged for review or canceled depending on the severity of the fraud score.
Fraud investigations
Artificial intelligence can scan hundreds of thousands of loan documents in real-time and give a list of flagged loan applications that a loan officer can investigate further.
This saves digital lending platforms thousands of man-hours that would have otherwise been spent finding fraudulent loan documents.
Purchase profiles
The system creates multiple purchase profiles by understanding user behaviour, allowing financial organizations to segment customers into profiles and monitor transactions.
KYC
AI-based KYC helps verify IDs and match fingerprints before authorizing any loans.
4. AI in Collection Management
Financial institutions analyze the bank transactions of at-risk accounts to identify customers who might default on loan repayment. AI enables lenders to take proactive measures and create personalized loan collection strategies for these customers.
For example, lenders can use targeted communications, payment reminders, and customized repayment plans for each borrower. They can set up chatbots to automate customer interactions and provide 24/7 support. On top of this, AI phone agents can conduct personalized outreach calls, efficiently addressing borrower concerns and facilitating timely repayments.
Together, these solutions reduce default rates, improve collection management, and improve customer satisfaction levels through loan management automation.
Impact of AI on Lending and Loan Management
The overall impact of using artificial intelligence-powered document processing tools can be condensed into two factors: increased accuracy and reduced processing speeds.
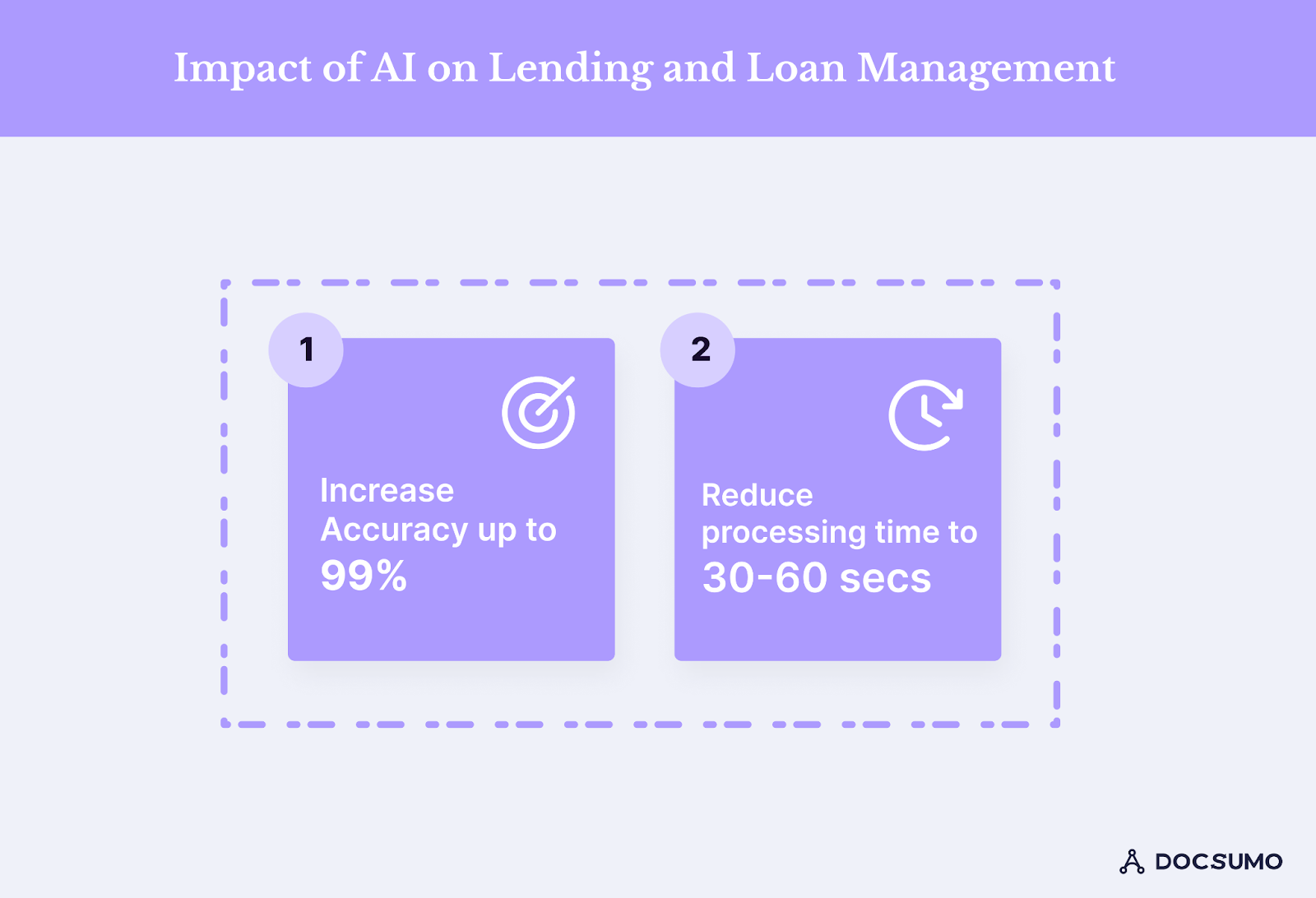
1. Increase accuracy rate to 99%
Accurate fraud detection and risk assessment, using AI, help lenders calculate precise loan amounts and avoid potential losses in fraud. For instance, the U.S. Department of the Treasury recovered nearly $375 million in 2023 due to its implementation of an enhanced fraud detection process that utilizes AI.
Digital lending platforms use advanced machine learning algorithms to analyze massive amounts of data to identify borrowing trends. Learning lending patterns helps banks enable more precise risk assessment and optimize their credit scoring system.
Implementing automated document processing leads to 99% accurate data extraction from structured and unstructured documents while eliminating human-prone errors. Integrating third-party systems and business workflows helps lenders streamline the loan application process.
2. Reduce processing time to 30-60 seconds
According to the Fannie Mae Mortgage Lender Sentiment Survey Report 2023, 73% of lenders reported improving operational efficiency as the primary motivation behind adopting AI/ML into the workflows.
Advanced and intelligent document processing systems extract specific information from financial statements, loan applications, and other supporting documents in seconds compared to traditional extraction methods that take days to weeks and several verification rounds.
Moving further, machine learning underwriting can assess creditworthiness and make precise risk assessments for faster loan approval and reduced loan processing time.
Check out how National Debt Relief writes off 350K Debt Settlement Letters yearly 10x faster
5 Challenges of Adopting AI in Lending and Loan Management
We know that AI is only as powerful as the data sets that are available to it. However, data leakage is a common concern for users when they share sensitive financial data. For organizations using AI lending, a user data breach could make them vulnerable to litigation and reputational damage.
Let’s look at the top challenges faced by financial institutions implementing artificial intelligence in their workflows.
1. Data privacy and security
Data algorithms must be privy to sensitive banking information to forecast trends and analyze credit risk effectively. Whatever software you implement for automating loan management, ensure that it is compliant with GDPR, SOC-2, CCPA, and regional regulatory requirements.
2. Bias and fairness concerns
The software relies on historical data to learn patterns, which might perpetuate biases and unfair treatment in the lending industry. Suppose the historical data contains any biases towards a specific racial group or section of society. In that case, the bias will continue unchecked through the system, and algorithms do not understand the contextual factors that might affect the borrower's creditworthiness.
Diverse data sets, transparent AI models, and rigorous algorithm testing in loan management automation address these challenges and promote fair lending practices.
3. Lack of transparency and interoperability
Lack of transparency in AI algorithms reduces trust and accountability in lending services. Therefore, human oversight of these algorithms and incorporating transparent reporting are critical to ensuring the integrity and reliability of financial services.
Additionally, the AI software might struggle to integrate with existing legacy systems, leading to data silos and inaccurate fraud detection. For instance, 56% of mortgage lenders consider integrating AI/ML systems with their existing infrastructure as their top challenge. Choosing software with robust integration capabilities is the key to seamlessly incorporating AI into loan management workflows.
4. Limited availability of data
Lenders and underwriters must train AI/ML models with vast amounts of quality data to improve performance and accurately detect fraud. However, most lenders need more accurate and complete data to help AI models strengthen their capabilities.
5. Implementation costs
Another significant challenge impacting AI adoption in lending is implementation and recurring costs. Incorporating AI loan management workflows can be expensive, considering the software, hardware, maintenance, and ongoing costs. Businesses must calculate the ROI and choose the solution that fits the budget.

How Does Docsumo Streamline Lending and Loan Management Processes?
Lenders and underwriters can leverage document processing AI to become more agile, efficient, and customer-centric. They can offer loans to deserving candidates, combat fraud, and prevent financial losses. However, understanding machine learning solutions, model deployment issues, and costs are some challenges stopping lending institutions from exploring the full potential of AI document processing.
Docsumo, an Intelligent Document Processing (IDP) platform, overcomes these limitations with its easy-to-use interface and quick deployment capabilities. The platform analyzes the borrower's financial documents and historical data to evaluate credit history, income, employment stability, debt-to-income ratio, and market conditions to flag potential risks.
Moreover, it validates extracted data with government databases to ensure authenticity. Therefore, underwriters and lenders only need to review exceptions, significantly reducing manual errors, enhancing customer experience, and improving efficiency.
Ready to reduce operational costs by 60-70% and process loan documents in 30-60 seconds?
Sign up for a free trial of Docsumo to efficiently mitigate fraud risks.
FAQs
1. What is AI lending?
AI is revolutionizing the lending industry, automating processes, reducing costs, and enhancing customer experiences.